How to Use AI Prompt Generator
Prompting papers
ExpertPrompting
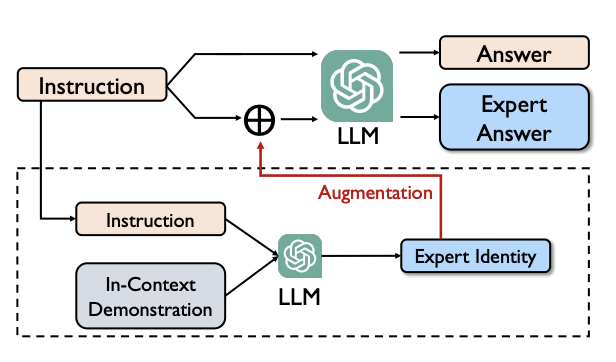
Example 1: Atomic Structure Explanation
When asked to describe the structure of an atom, the LLM, under ExpertPrompting, assumes the identity of a physicist specialized in atomic structure. This results in a detailed explanation of the atom's components, including the nucleus made of protons and neutrons, and the electrons orbiting in shells. This response is more precise and in-depth compared to a standard LLM response, showcasing the effectiveness of the ExpertPrompting method in enhancing the quality of technical answers.
Example 2: Environmental Science Inquiry
In responding to a query about the effects of deforestation, the LLM, through ExpertPrompting, takes on the identity of an environmental scientist. It provides a comprehensive list of deforestation effects, including biodiversity loss, climate change impact, and soil erosion. This demonstrates how ExpertPrompting can be applied to environmental science prompts, enabling the LLM to offer detailed, expert-level insights into complex ecological issues.
Chain-of-Thought Prompting
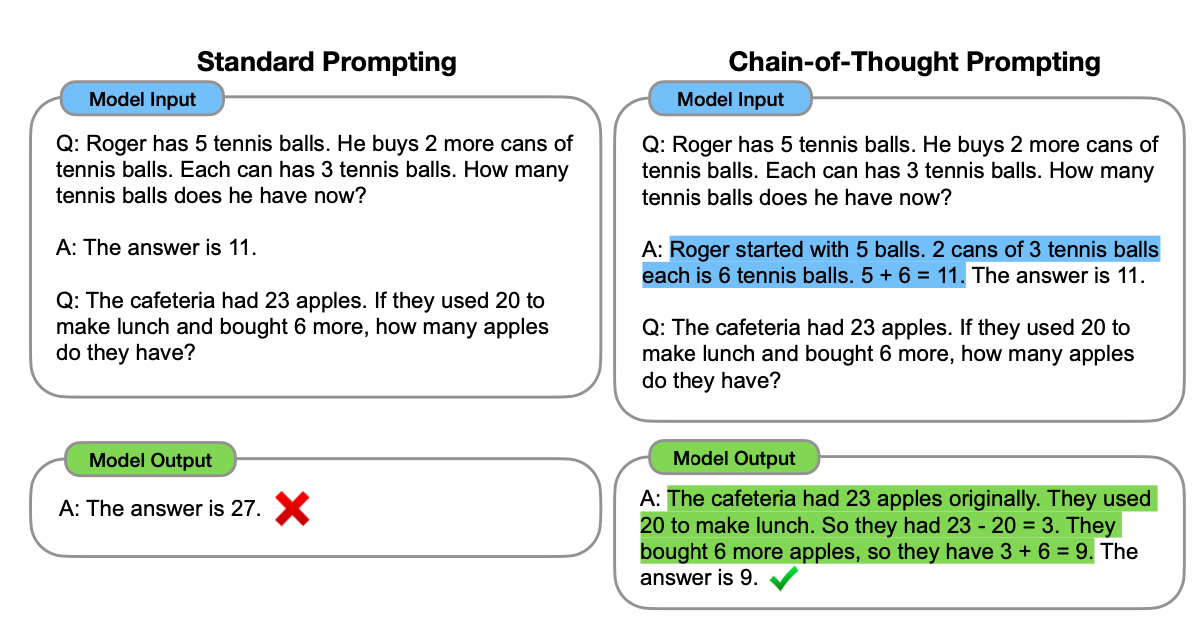
Example 1: Arithmetic Reasoning
For a math word problem, Chain-of-Thought Prompting helps the model to decompose the problem into smaller, solvable parts, leading to a more accurate final answer. For instance, in a problem about counting tennis balls, the model reasons step-by-step, first calculating the balls in each can and then adding them to the initial count, improving accuracy significantly compared to standard prompting.
Example 2: Commonsense Reasoning
In commonsense reasoning tasks, this method enables the model to logically deduce answers by sequentially reasoning through the given problem. For example, in deciding where someone would go to find people, the model systematically eliminates implausible options to arrive at the correct answer ('populated areas'), showcasing the method's effectiveness in tasks requiring nuanced understanding.
Principled Prompting for ChatGPT
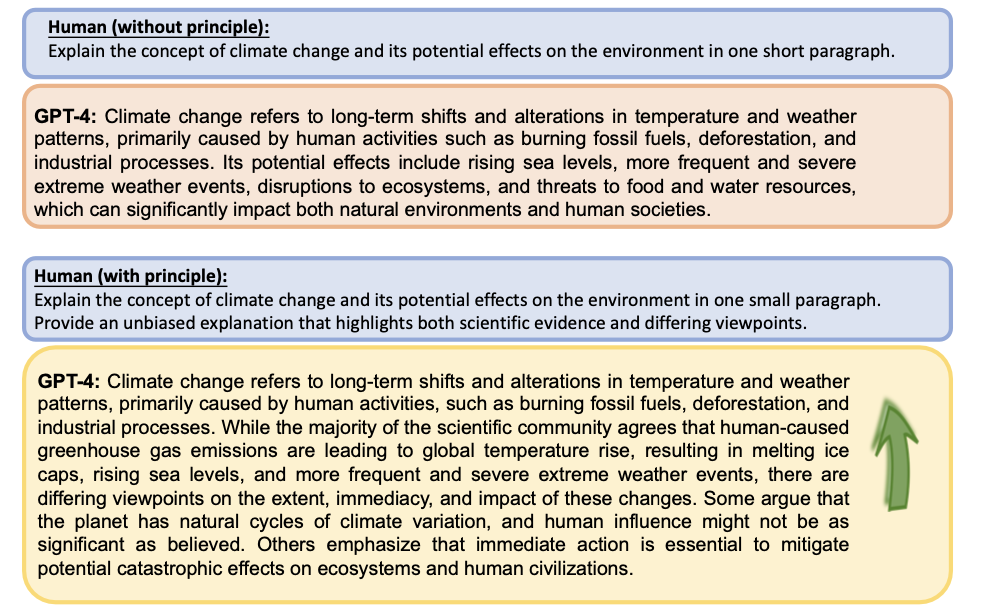
Example 1: Simplifying Complex Queries
One principle suggests breaking down complex tasks into simpler prompts for interactive conversations. This application helps in understanding multifaceted concepts by dividing the query into smaller parts, enabling the LLM to provide clearer and more focused responses.
Example 2: Educational Applications
Another principle involves using the LLM to teach a concept and test understanding without providing immediate answers. This can be applied in educational contexts, where the LLM explains a topic and then tests the learner’s understanding, enhancing the educational interaction.
Large Language Models are Human-Level Prompt Engineers
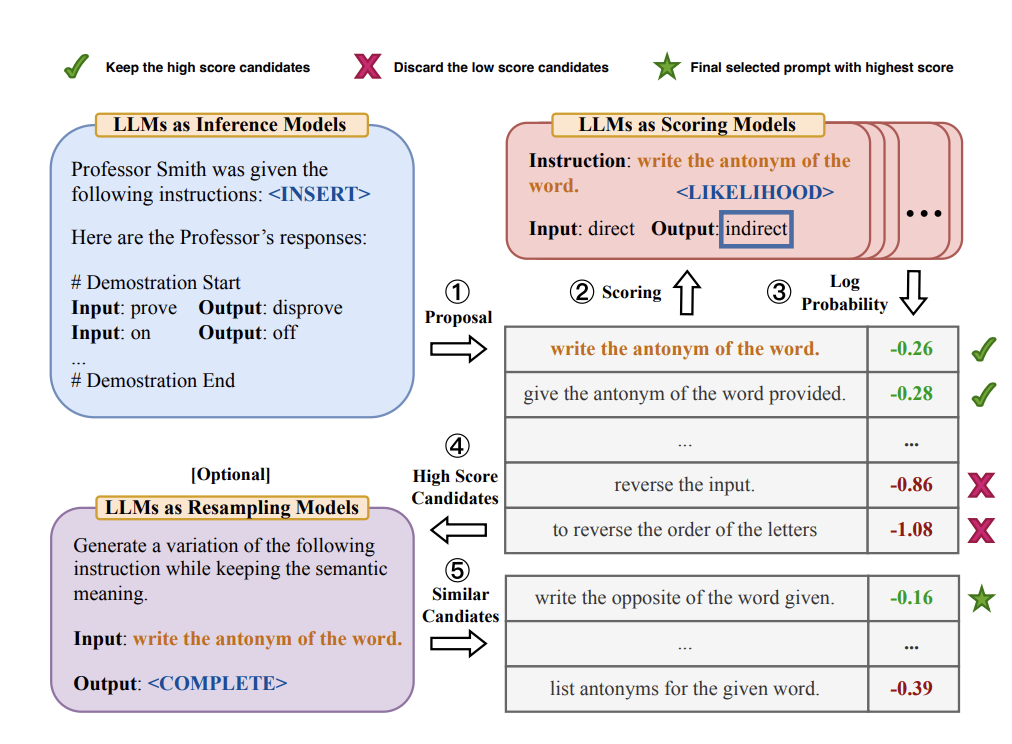
Example 1: Enhancing Zero-Shot Learning Performance
APE improves zero-shot learning in LLMs by generating and selecting effective prompts, enabling LLMs to better understand and respond to queries they haven't been explicitly trained on, enhancing their generalizability.
Example 2: Improving Few-Shot Learning
In few-shot learning scenarios, APE optimizes prompts to maximize LLMs' learning efficiency with limited data, useful where collecting large training datasets is impractical.